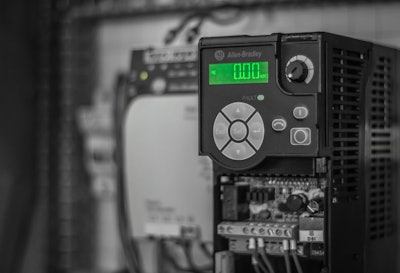
As artificial intelligence (AI) continues to revolutionize industries worldwide, the commercial grain handling sector is on the brink of a digital transformation. From predictive maintenance to automated decision-making, AI-driven solutions are enhancing operational efficiency, security and data management in grain elevators. At a GEAPS Exchange 2025 concurrent session, Dave Smit, OT architect for Interstates, explored the role of AI in grain facilities, the critical steps for infrastructure readiness, and the importance of data governance in maximizing AI’s potential.
The digital transformation journey in grain facilities
Smit said that before grain facilities can fully leverage AI, they must first ensure they have a robust digital infrastructure in place.
“AI is not the starting place,” Smit said. “AI is the next step. The whole digital transformation is the starting place.”
AI adoption in grain handling follows a structured progression through five stages of digital transformation:
- Standard Reporting – Traditional grain elevators have relied on paper-based systems for data tracking and reporting.
- Descriptive Analytics – With digitalization, data is now captured and stored centrally, allowing manual analysis.
- Diagnostic Analytics – Automation helps identify trends and informs decision-making for operational improvements.
- Predictive Analytics – AI connects data from multiple departments, enabling proactive maintenance and resource allocation.
- Prescriptive Analytics – Advanced AI solutions integrate analytics and intelligence to automate complex decision-making.
Infrastructure readiness for AI
Smit said that to effectively implement AI, grain facilities must establish a strong digital foundation. Preparing for AI integration involves several critical steps:
- Network assessment: Evaluating existing infrastructure for AI readiness.
- Invest in scalable and secure hardware and software: Future-proofing systems with flexible, upgradeable solutions.
- Collaborate with vendors and experts: Leveraging industry expertise to implement best practices and emerging technologies.
- Develop a roadmap: Defining short-term and long-term AI implementation goals.
- Evaluate emerging AI technologies: Staying updated on AI advancements relevant to grain handling operations.
- Train personnel: Ensuring employees are equipped with the skills to manage and utilize AI tools effectively.
Smit highlighted the need for modernized infrastructure.
"Fifty years ago, all our networks were isolated,” he said. “We didn't even have Ethernet. Nobody could get to our networks. Now, as we start to apply these new technologies, our networks are becoming exposed, and cybersecurity is becoming a real issue.”
AI-driven security integration
Security is a major concern in the digital transformation of grain facilities. AI-driven security solutions help mitigate risks associated with cyber threats, data breaches and operational vulnerabilities. Key threats include:
- Phishing and social engineering attacks
- Legacy systems and technical debt
- IoT and OT vulnerabilities
- Ransomware and malware
- Third-party and supply chain risks
- Data privacy and compliance risks
“Everybody knows that cybersecurity threats in OT are on the rise,” Smit warned. “It's no longer just some geeky dude in his basement wearing a hoodie trying to attack you. They now have sophisticated systems and they’re using AI as tools to do this all.”
Predictive maintenance advancements
One of AI’s most impactful applications in grain handling is predictive maintenance. By analyzing real-time sensor data and historical performance metrics, AI can anticipate equipment failures before they occur. Benefits of AI-driven predictive maintenance include:
- Reduced downtime: Early failure detection enables proactive maintenance.
- Lower maintenance costs: Addressing issues before they escalate.
- Extended equipment lifespan: Optimizing machinery performance.
- Improved safety: Preventing hazardous equipment malfunctions.
The value of predictive analytics lies in being able to plan for downtime instead of reacting to unexpected downtime.
“Take a bearing, for example,” Smit said. “We know that after X number of hours on a bearing, it's going to fail at a certain time because we're monitoring that and we can replace that bearing before it fails."
Operational efficiency improvements
AI enhances operational efficiency by automating complex processes and optimizing workflows. Some key AI-driven efficiency solutions include:
- Automating decision-making processes
- Real-time grain storage allocation
- Workflow optimization
- Piloting AI applications in high-impact areas
Smit described what the future might look like at a grain elevator at the peak season of harvest.
“Everybody’s busy, there's dust in the air and trucks are lined up everywhere — all hands are on deck,” Smit said. “Now imagine if AI was managing all that. AI was doing all the receiving for you. AI told the trucks where to go because it's optimized the whole system.”
The importance of data governance
AI’s effectiveness is only as strong as the data that fuels it. Poor data governance can introduce significant risks, including inaccurate predictions and unreliable automation. Establishing a strong data governance framework ensures AI operates efficiently and securely.
Building a data governance framework
- Define clear data standards and quality metrics
- Establish ownership and accountability
- Regularly audit and update data sets
- Identify and prioritize key data sources
- Implement secure data storage and access controls
- Train staff on data management best practices
Smit underscored the importance of data governance.
"AI models are only as good as the data that we’ve trained them with,” he said. “If we don’t have any good data, we can’t train them well. So it’s really important to start collecting that data today so that maybe in two or three years when you’re ready for these solutions, you have two or three years of data that you can apply.”
The future of AI in grain handling
AI is transforming the grain industry, providing unprecedented levels of automation, security and operational efficiency. Facilities that embrace digital transformation and prioritize data governance will be best positioned to capitalize on AI’s full potential. By investing in the right infrastructure and security measures, grain elevators can enhance productivity, reduce risks and drive long-term profitability in an increasingly competitive industry.
As AI adoption accelerates, organizations must prepare now.
“It's not a matter of ‘are we going to be able to?'” Smit said. “These technologies are coming, and we have to be ready for them, We’re not going to be able to do what we've done for the last 50 years. We have to make some changes to be able to support this, or else we're going to have issues.”
Long-term success depends on whether grain facilities will take the necessary steps to integrate it effectively and stay ahead of the curve.